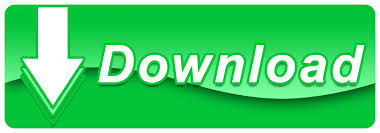
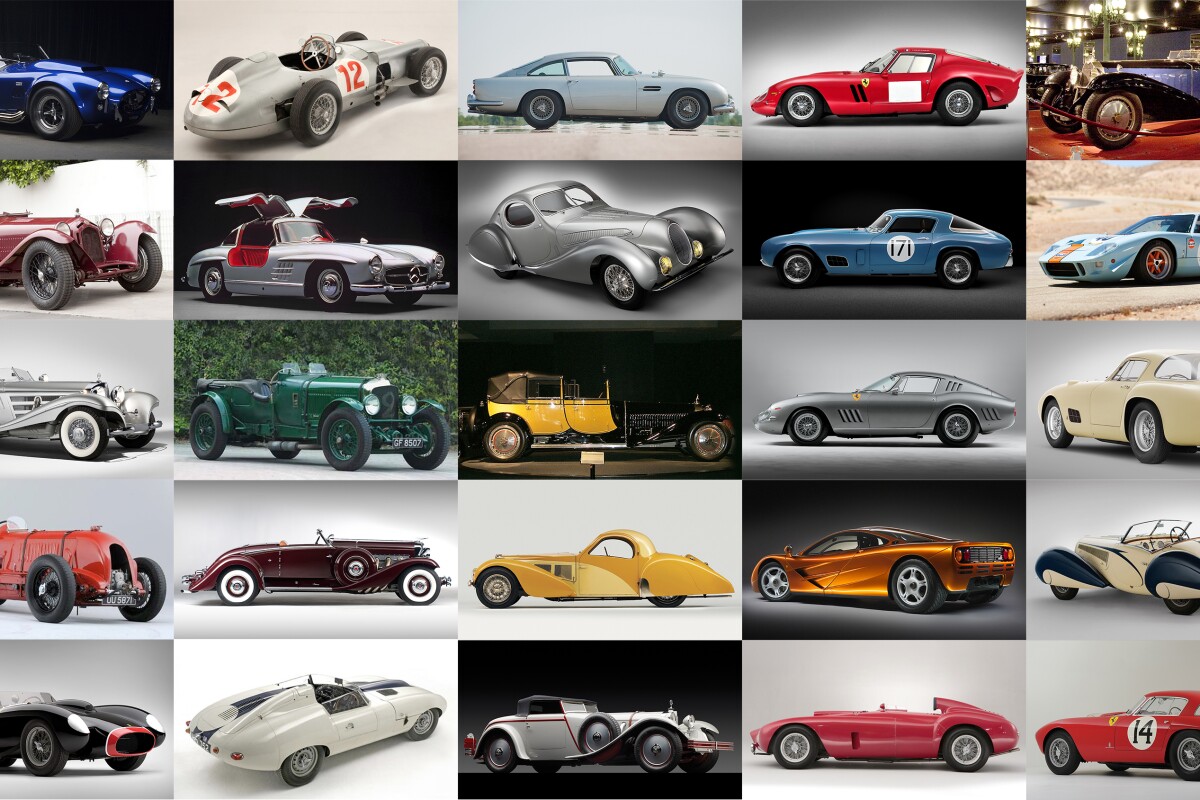
However, most real networks compose of different types of nodes which opened a new research topic called as heterogeneous networks. An excellent survey on these categories can be found in ref. The posterior probabilities are obtained by defining a conditional probability model over the learned parameters. The main idea is to optimize a target function in order to establish a parametric model that can best fit the observed data. The third category is probabilistic based methods. Then, any conventional supervised learning algorithms might be applied to train a supervised link predictor 9. Any similarity indices mentioned in the previous category could form the required feature vectors. In this regard, a feature vector is extracted for each node pair and a 0/1 label would be assigned based on the existence/not-existence of that link in the network. In the second category, the link prediction is defined as a two-class classification problem. This approach has been also successfully extended on the bipartite complex networks 8. It has been demonstrated through extensive experimental evaluations that LCP based indices could provide better performance predictions compared to other conventional indices. The authors proposed Cannistraci variations of CN, JC, AA, RA, and PA called as CAR, CJC, CAA, CRA, and CPA. Accordingly, two nodes are more likely to be connected if they have some common neighbors belonging to a densely formed local community. Recently, the integration of both node and link based topological information has been studied by introducing local community paradigm (LCP) 7. While the local indices are simple in computation, the global ones may provide more accurate predictions. Common Neighbors (CN), Jaccard (JC), Prefrential Attachment (PA), Adamic Adar (AA), and Resource Allocation (RA) are among popular local indices, while Katz, Leicht-Holme-Newman, Average Commute Time, Random Walk, and SimRank are known as global indices 6. In the first category, the aim is to extract some local (node-based) or global (path-based) similarity features for vertices or links. They are divided into some categories as local/global similarity indices, supervised, and probabilistic methods. The majority of link prediction approaches have been proposed on homogenous complex networks. It has many real-world applications like friend recommendation in social networks 2, detecting selfish or spurious nodes/edges in social networks 3, citation predicting in scientific collaboration networks 4, modeling the evolution of complex networks 5, etc. The aim of link prediction is to exploit dependencies between any node pairs 1.
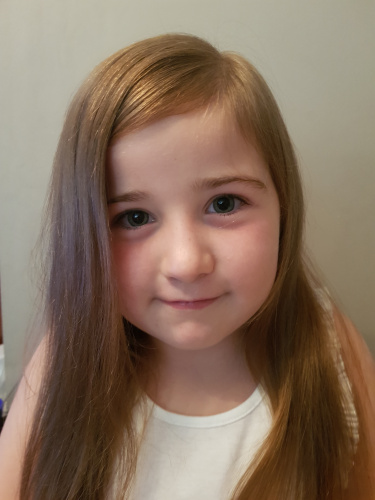
Link prediction is an interesting research area in complex networks. The experimental results on a Bibliography network show that the MMI obtains high prediction accuracy compared with other popular similarity indices. This estimation measures the amount of information through the paths instead of measuring the amount of connectivity between the node pairs. The proposed model, called as Meta-path based Mutual Information Index (MMI), introduces meta-path based link entropy to estimate the link likelihood and could be carried on a set of available meta-paths. To tackle with these problems, we propose a mutual information model for link prediction in heterogeneous complex networks. Hence, employing a set of different meta-paths is not straightforward. Secondly, most of them are required to use a single and usually symmetric meta-path in advance. Firstly, they are primarily dependent on the connectivity degrees of node pairs without considering the further information provided by the given meta-path. However, these indices suffer from two major drawbacks. Recently, a number of meta-path based similarity indices like PathSim, HeteSim, and random walk have been proposed for link prediction in heterogeneous complex networks.
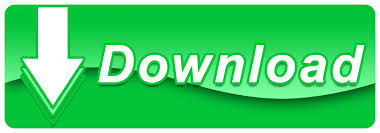